Currently Empty: £0.00
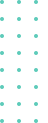
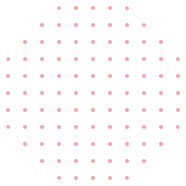
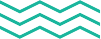
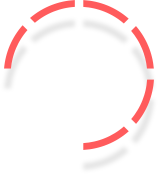
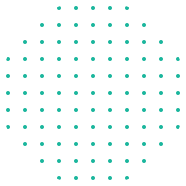
Instructor

200+
Instructors
Instructors
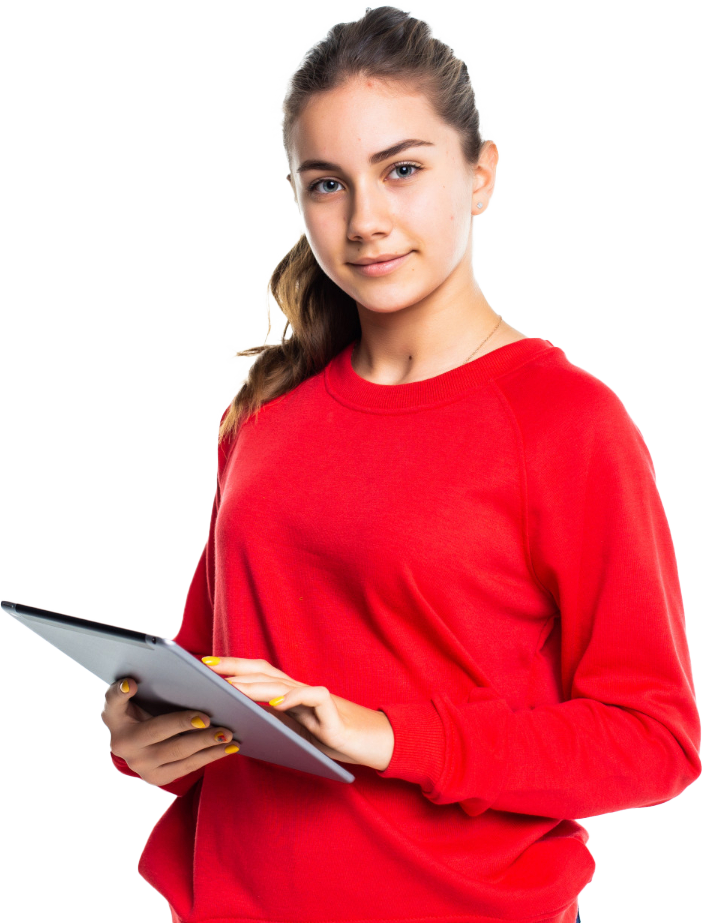
60
Online Courses
Top
Instructors
Online
Certifications
2,000
Membership
All Categories Courses
Latest Technology Training Courses & Certifications Online
Training.
Discover the future of career shaping with LSA Training and HCL Tech.
Are you ready to bridge the global technology skills gap and stay ahead in the ever-evolving tech industry? Look no further! LSA Training and HCL Technologies have joined forces to bring you the ultimate job readiness training program.
With our cutting-edge curriculum and expert instructors, we are committed to equipping you with the latest technologies and ensuring you have the skills needed to excel in the digital world. Our instructor-led online training provides a flexible and immersive learning experience, allowing you to learn at your own pace while still benefiting from personalized guidance.
Whether you're a seasoned professional looking to upskill or a fresh graduate eager to kick-start your career, our partnership offers a comprehensive range of courses that cater to all skill levels. From AI and machine learning to cloud computing and cybersecurity, we cover it all!
Don't miss out on this incredible opportunity to boost your tech prowess and gain a competitive edge in the job market. Join LSA Training and HCL Technologies today and unlock a world of exciting career possibilities.
Sign up now at https://www.lsatraining.co.uk/en-in/ embark on your journey towards success!
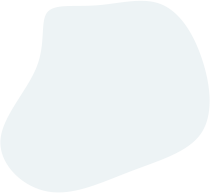
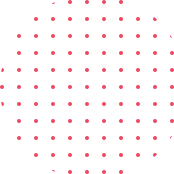
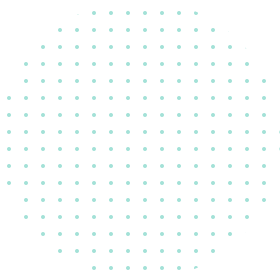
A Collaboration Between
LSA Training and HCL Tech
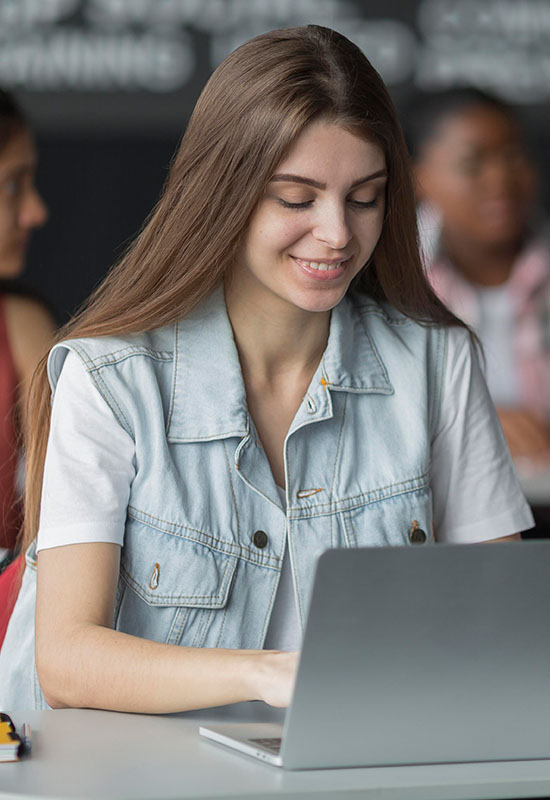
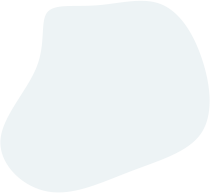
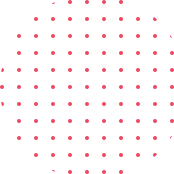
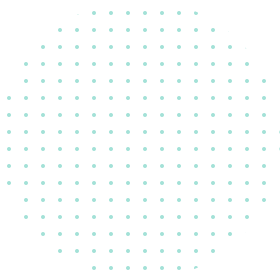
29+
Wonderful Awards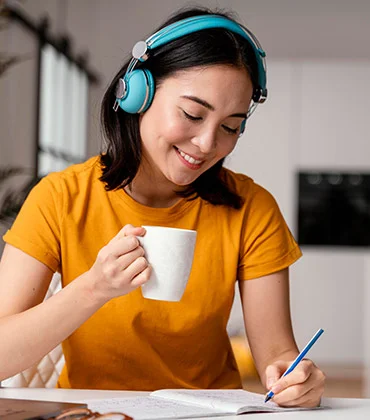
ABOUT US
Unique things about LSA Training
Welcome to LSA Training , a premier software training centre that offers a wide range of courses in the latest technologies and trends. Our training centre is designed to help professionals gain the skills and knowledge they need to succeed in today’s dynamic and competitive job market.
At LSA Training, we offer specialized training courses in AWS/DevOps, Cyber Security, Software Testing, and Data Analyst. Our courses are designed and delivered by industry experts with years of experience in their respective fields. We believe in a hands-on approach to learning, where students get practical experience in real-world scenarios that they will encounter in their jobs.
- Expert Trainers
- Online Remote Learning
- Lifetime Access
POPULAR COURSES
Pick A Course To Get Started
expert
(0.0/ 0 Rating)
Data Engineer – advanced
£1,500.00
Welcome to Data Engineering- Advanced learning in career shaper. We have curated the content to enhance your competency as a...
- 0 Lessons
- 30 Students
All Levels
(0.0/ 0 Rating)
D365 Analyst
£1,500.00
Welcome to D365 learning in career shaper We have curated the content to enhance your competency as a D365 Analyst...
- 0 Lessons
- 30 Students
All Levels
(0.0/ 0 Rating)
Cassandra DBA
£1,500.00
Course Description Course Content Highlights Course Description Welcome to Cassandra learning in career shaper Welcome to Cassandra learning in career…
- 0 Lessons
- 31 Students
All Levels
(0.0/ 0 Rating)
Snowflake Developer
£1,500.00
Welcome to Snowflake learning in career shaper We have curated the content to enhance your competency as a Snowflake Developer...
- 0 Lessons
- 30 Students
It’s worth noting that 25% of the top-performing candidates identified through this program will have the opportunity to join HCL Tech, while participants will also receive certifications recognized globally, enhancing their professional credentials.
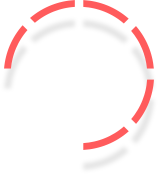
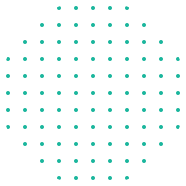
CENTRES
+
YEARS IN BUSINESS
+
ACTIVE COURSES
+
PROFESSIONALS TRAINED
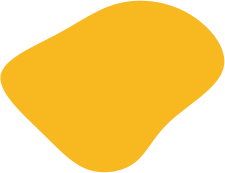
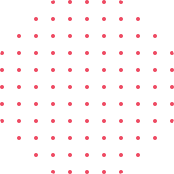
TESTIMONIALS
What Our Students Have To Say
The customer’s perception is your reality
I recently attended an Automation Testing course with them. The experience so far is really good. The course structure was quite clear and easy to follow. LSA helped me to be more confident and kept me updated with my knowledge that I needed to surface in the job market. The course structure also demands lot of effort and practise from one's side. The tutor and the management team are very helpful. For people who wants an entry into IT testing, LSA is the place to stop.
Priya darsini
I would 100% recommend LSA training who wants to pursue their career in Microsoft Azure. Excellent teaching A very inspirational course They make us understand all the core concepts in an easy and simple way. Excellent centre, wonderful management and a very helpful tutors full services.
bobbily bhagath
I attended manual and automation testing training with LSA. The training centre is brilliant! They took me from zero level to an expert level. I have secured a testing role through their testing training.I can recommend the centre for any newbies or to update your knowledge about software testing.
Richard Davis
Had amazing experience partaking in Manual/Automation training. Venu is a great tutor and attention to detail, he is always very supportive and ready to answer your questions and explain things to you thoroughly. After the training, you will also get support from the Director (Mr Gopal) which will guide you through the process applying for jobs in Software Testing. HIGHLY RECOMMENDED. A++++++
Tee JaY
OUR PARTNERS
A Few of Our Clients
Success is the sum of small efforts, repeated day in and day out.
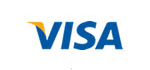
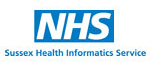
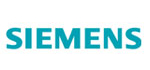
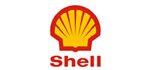
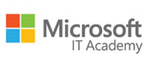
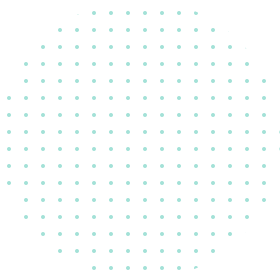
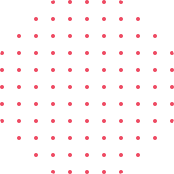